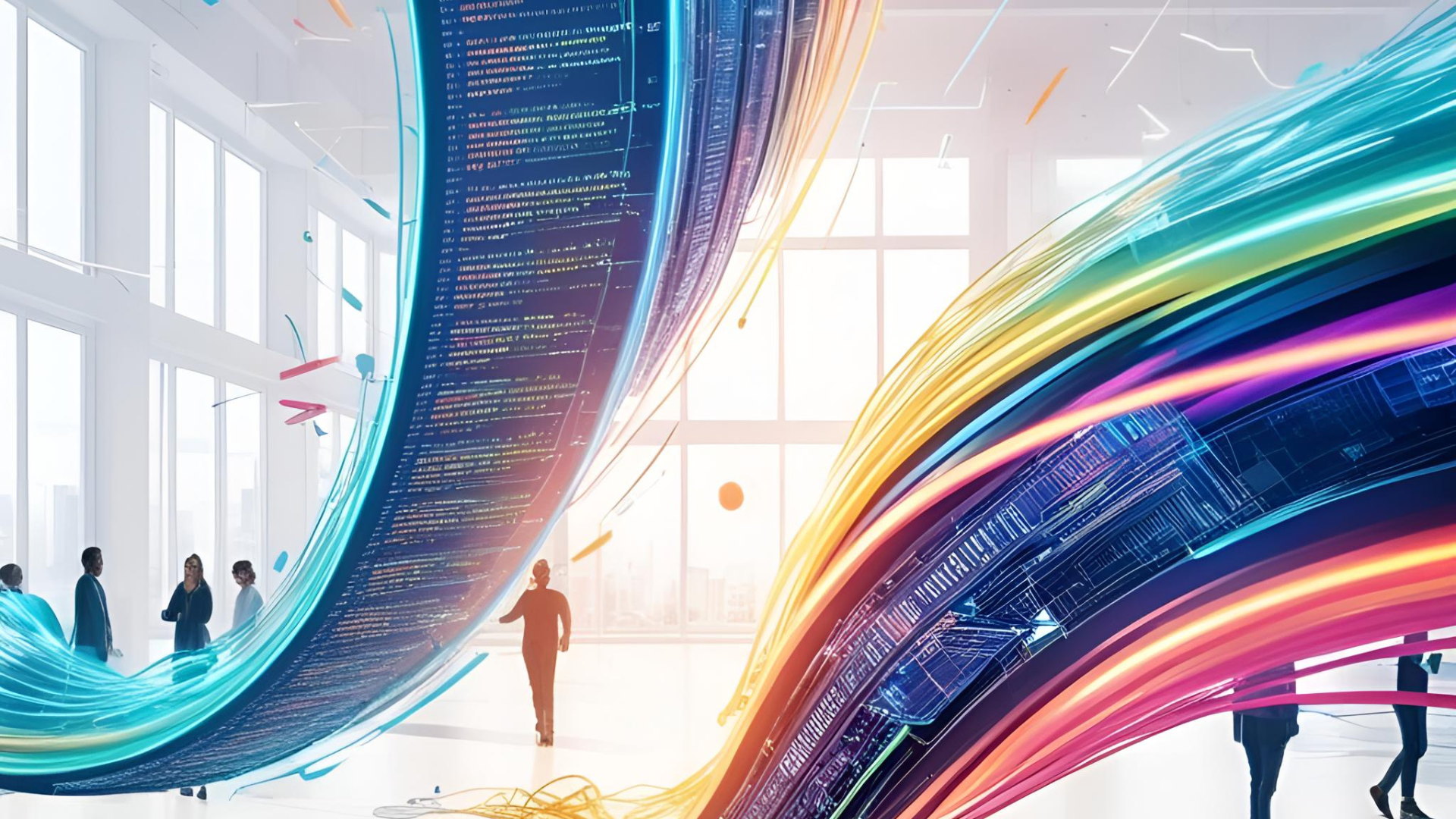
Every enterprise operates with some degree of technical debt. With millions of lines of legacy code powering critical operations, technical debt is inevitable—eventually, all code becomes outdated. While technical debt isn’t inherently “bad,” it can limit an enterprise’s ability to adapt, innovate, and remain competitive.
Addressing technical debt isn’t just about identifying issues; it’s about managing them across an enterprise modernization initiative with orchestration, insight, and accountability. That’s where platforms like MLP (Modernization Lifecycle Platform) become essential.
AI-powered modernization solutions offer advanced capabilities to enhance the assessment and management of technical debt, such as:
Automated Code Analysis
AI-powered tools can automatically analyze codebases to detect code smells, architectural issues, and other indicators of technical debt. For example, CodeScene uses machine learning algorithms to identify patterns in version control data, highlighting hotspots, i.e., code areas frequently modified and may require attention. This behavioral code analysis helps prioritize technical debt mitigation efforts.
Predictive Maintenance
AI can predict which parts of the code will likely cause future issues by analyzing historical data and code evolution patterns. This foresight enables teams to proactively address potential problems before they escalate, effectively managing technical debt.
Prioritization of Refactoring Efforts
AI can assess the impact of technical debt on various aspects of software performance and maintainability, helping teams prioritize refactoring efforts based on factors like code complexity, defect density, and contribution to business goals. Tools like NDepend provide metrics and visualizations that assist in understanding and managing technical debt within .NET applications.
Estimation of Remediation Costs
AI can estimate the effort required to address specific technical debt items, enabling better planning and resource allocation. The SQALE method, for instance, offers a framework for assessing source code quality and estimating the remediation costs associated with technical debt.
Continuous Monitoring and Reporting
AI-driven tools can continuously monitor codebases for new technical debt instances, providing developers real-time feedback. This continuous integration ensures that technical debt is managed proactively, preventing its accumulation over time.
These AI capabilities are most effective when deployed within a unified modernization framework. MLP provides the end-to-end infrastructure to integrate AI into each phase of technical debt remediation, from initial discovery and impact assessment to automated code transformation and final validation. By embedding AI tooling into the MLP workflow, organizations can move beyond static analysis to execute modernization plans with measurable outcomes and full traceability.
Platforms like MLP make this integration actionable by supporting AI-assisted analysis and rule-based automation across diverse environments, including mainframe, midrange, and distributed systems. MLP’s ability to coordinate modernization assets, automate repetitive tasks, and generate audit trails gives enterprises a practical path to address technical debt while aligning modernization efforts with business priorities.
By integrating AI into the software development lifecycle, organizations can significantly enhance their ability to identify, assess, and manage technical debt. This will lead to more maintainable codebases, efficient development processes, and a stronger foundation for future innovation.
Related Posts: